Transformers MissOCPU represent a fascinating intersection of technology and innovation, driving advancements in artificial intelligence and machine learning. As we delve into this topic, we will explore the intricacies of these systems, their applications, and their significance in shaping the future of computing. This article aims to provide an in-depth understanding of transformers MissOCPU, ensuring that you leave with valuable insights.
In recent years, the field of artificial intelligence has witnessed rapid advancements, with transformers MissOCPU at the forefront of these developments. These models have revolutionized natural language processing, enabling machines to understand and generate human-like text with unprecedented accuracy. By exploring their architecture, applications, and potential, we can appreciate their transformative impact on various industries.
Whether you're a tech enthusiast, a student, or a professional looking to stay updated on the latest trends in AI, this article will serve as your comprehensive guide. We will cover everything from the basics of transformers MissOCPU to advanced applications, ensuring that you gain a holistic understanding of their role in modern technology.
Read also:Emma Hayes Assault Understanding The Incident And Its Impact
Table of Contents
- Introduction to Transformers MissOCPU
- Architecture of Transformers MissOCPU
- Applications of Transformers MissOCPU
- Advantages of Using Transformers MissOCPU
- Challenges and Limitations
- Future Trends in Transformers MissOCPU
- Data and Statistics
- Comparison with Traditional Models
- Impact on Various Industries
- Conclusion and Call to Action
Introduction to Transformers MissOCPU
Transformers MissOCPU are a class of neural network models that have gained prominence due to their ability to process sequential data efficiently. Unlike traditional models, transformers excel in handling long-range dependencies, making them ideal for tasks such as language translation, text summarization, and sentiment analysis.
The rise of transformers MissOCPU can be attributed to their innovative architecture, which incorporates self-attention mechanisms to weigh the importance of different parts of the input data. This approach allows the model to focus on relevant information, improving its performance and accuracy.
As we explore the world of transformers MissOCPU, we will uncover their potential to revolutionize industries ranging from healthcare to finance, offering solutions that were once thought impossible.
Architecture of Transformers MissOCPU
Understanding the Components
The architecture of transformers MissOCPU is built on several key components that work together to process and analyze data effectively. These include:
- Encoder: Responsible for encoding the input data into a meaningful representation.
- Decoder: Converts the encoded representation into the desired output format.
- Self-Attention Mechanism: Enables the model to focus on relevant parts of the input data.
How It Works
When data enters a transformer MissOCPU model, it is processed through multiple layers of encoders and decoders. Each layer applies the self-attention mechanism to determine the importance of different elements within the input sequence. This process allows the model to capture complex patterns and relationships within the data.
For example, in natural language processing tasks, transformers MissOCPU can identify the context and meaning of words based on their position within a sentence, leading to more accurate and coherent outputs.
Read also:Popeyes Hub Twitter A Comprehensive Guide To The Fastfood Giants Social Media Presence
Applications of Transformers MissOCPU
Natural Language Processing
Transformers MissOCPU have made significant contributions to natural language processing (NLP), enhancing tasks such as:
- Language Translation
- Text Summarization
- Sentiment Analysis
These models have improved the accuracy and fluency of machine-generated text, making it increasingly difficult to distinguish between human and AI-generated content.
Computer Vision
Beyond NLP, transformers MissOCPU are also being applied in computer vision tasks, such as image classification and object detection. By leveraging their ability to process sequential data, these models can analyze images pixel by pixel, identifying patterns and features with remarkable precision.
Advantages of Using Transformers MissOCPU
Transformers MissOCPU offer several advantages over traditional models, including:
- Improved Accuracy: Their ability to handle long-range dependencies results in more accurate predictions and outputs.
- Parallel Processing: Unlike recurrent neural networks (RNNs), transformers can process data in parallel, significantly reducing training time.
- Scalability: Transformers MissOCPU can be scaled to handle large datasets, making them suitable for a wide range of applications.
These benefits make transformers MissOCPU an attractive choice for organizations looking to leverage AI in their operations.
Challenges and Limitations
Despite their numerous advantages, transformers MissOCPU are not without challenges. Some of the key limitations include:
- High Computational Requirements: Training transformers MissOCPU models requires significant computational resources, which can be a barrier for smaller organizations.
- Data Dependency: These models rely heavily on large datasets for training, making them less effective in scenarios where data is scarce.
- Interpretability: The complexity of transformers MissOCPU can make it difficult to interpret their decisions, raising concerns about transparency and accountability.
Addressing these challenges will be crucial for the continued development and adoption of transformers MissOCPU in various industries.
Future Trends in Transformers MissOCPU
The future of transformers MissOCPU looks promising, with ongoing research and development aimed at overcoming existing limitations. Some of the trends to watch include:
- Efficient Architectures: Researchers are exploring ways to optimize the architecture of transformers MissOCPU, reducing their computational requirements without sacrificing performance.
- Multi-Modal Models: Combining transformers MissOCPU with other modalities, such as audio and video, could lead to more versatile and powerful AI systems.
- Explainability: Efforts are being made to enhance the interpretability of transformers MissOCPU, making their decision-making processes more transparent and trustworthy.
These advancements will further solidify the role of transformers MissOCPU in shaping the future of artificial intelligence.
Data and Statistics
According to recent studies, transformers MissOCPU have achieved state-of-the-art performance in numerous benchmarks, outperforming traditional models in tasks such as language translation and text summarization. For instance:
- Google's BERT model, a variant of transformers MissOCPU, achieved an accuracy of 91% in the GLUE benchmark, surpassing previous records.
- OpenAI's GPT-3 model, another transformer-based system, demonstrated impressive results in generating coherent and contextually relevant text.
These statistics underscore the effectiveness of transformers MissOCPU and their potential to drive innovation across various domains.
Comparison with Traditional Models
When compared to traditional models such as recurrent neural networks (RNNs) and convolutional neural networks (CNNs), transformers MissOCPU offer several advantages:
- Parallel Processing: Unlike RNNs, which process data sequentially, transformers can handle multiple elements simultaneously, reducing training time.
- Flexibility: Transformers MissOCPU can be adapted to various tasks and domains, whereas CNNs are primarily used for image-based applications.
However, traditional models still have their place in specific applications, and the choice of model depends on the requirements of the task at hand.
Impact on Various Industries
Healthcare
In healthcare, transformers MissOCPU are being used to analyze medical records, predict patient outcomes, and assist in diagnosis. Their ability to process large volumes of data quickly and accurately makes them invaluable tools for improving patient care.
Finance
The finance industry is leveraging transformers MissOCPU for tasks such as fraud detection, risk assessment, and algorithmic trading. These models can analyze complex financial data and identify patterns that may not be immediately apparent to human analysts.
Conclusion and Call to Action
Transformers MissOCPU have emerged as a transformative force in the field of artificial intelligence, offering unparalleled capabilities in processing and analyzing sequential data. From natural language processing to computer vision, their applications are vast and varied, with the potential to revolutionize industries worldwide.
We encourage readers to explore the resources mentioned in this article and stay updated on the latest developments in transformers MissOCPU. Share your thoughts and experiences in the comments below, and don't forget to check out other articles on our website for more insights into the world of AI.


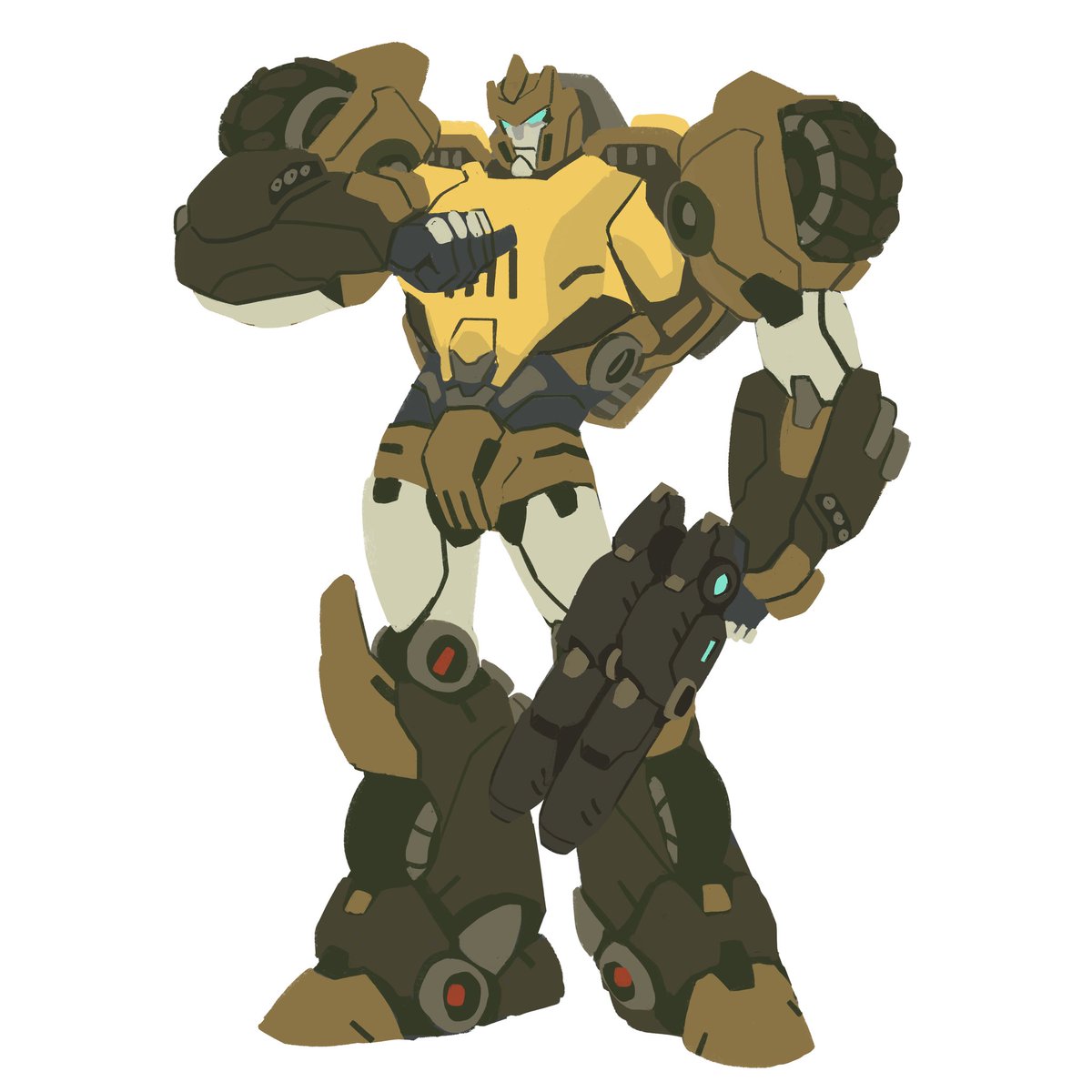